Applying the Enterprise Risk Mindset to AI
- Ana Hadnes Bruder,
- Arsen Kourinian,
- Oliver Yaros,
- Meghan Milloy,
- Matt Saidel
At A Glance
Artificial intelligence (AI) and other emerging technologies have the potential to revolutionize the financial industry. In fact, many financial services firms already use AI, though most organizations are in the early stages of adoption and integration. In our financial services report—The Next Organization: Seven Dimensions of a Successful Business Transformation—we note that more than seven in 10 leaders of financial institutions (71%) and over eight in 10 leaders of investment firms (83%) said that, in the next three years, pervasive AI will have a significant impact on the market environment. However, fewer than a third of these leaders believe they have a sufficiently clear and future-ready strategy in place for AI. Most of these leaders (72% of financial institution leaders and 73% of investment firm leaders) said AI is developing so fast that their organization is having difficulty adjusting quickly enough.
According to a survey by the European Securities and Markets Authority (ESMA), many credit rating agencies and market infrastructures, including data reporting service providers, already use generative AI (GenAI) tools or plan to start using them soon. Banks and financial institutions may use AI in their lending decision-making processes, and insurers may use AI to generate claims settlement offers.
While AI presents myriad opportunities to boost efficiency, productivity, and industry advancements, it also brings with it myriad risks. While these risks may not be new, the rapid acceleration and proliferation of AI has seen them intensify in unique ways:
- Organizations usually haven’t developed the AI tools they use, and may lack insight into how their tools may work, making it more challenging to understand and sidestep these risks.
- People are reportedly inherently more trusting of machines. Even though AI users don’t have insight into how a tool was created, human nature often compels them to trust AI results regardless of their reliability (referred to as “automation bias”). Organizations need to ensure meaningful human intervention into AI output, including critiquing, assessing, refining, and potentially overriding results.
- New laws are expanding existing requirements and creating new obligations. For instance, some provisions of the EU AI Act will soon take effect. Beginning February 2025, companies will be prohibited from using certain AI functions, such as:
- AI systems that use deceptive techniques, exploit vulnerabilities, or use social scoring, create or expand facial recognition databases through untargeted scraping of the internet or CCTV footage;
- or for predictive policing, inferring emotions in the workplace, using biometric data to categorize individuals, or running real-time remote biometric identification systems.
- The EU AI Act applies to AI systems used or developed in the European Union (EU), as well as whenever the output of its AI system is used in the EU (even if the organization using the tool is outside of the EU).
- In the United States, a similar comprehensive and risk-based AI law goes into effect in Colorado on February 1, 2026. Colorado’s AI law provides detailed obligations that developers and deployers are required to implement for high-risk AI systems in order to avoid algorithmic discrimination. Other states, such as Utah, Illinois, New York City, and California, have also passed lighter versions of AI laws focused on transparency and discrimination in employment risks. We anticipate more state-by-state AI laws to pass in the coming years in the absence of federal legislation, including legislation that mirrors the EU AI Act. Even the UK government—which has, up until now, not regulated in order to take a more “pro-innovation” approach—is now considering issuing legislation covering the use of AI following on from the launch of the UK’s AI Opportunities Action Plan in January 2025, in which the prime minister announced that the United Kingdom will pursue a pragmatic approach, testing AI systems in areas like healthcare and education in advance of adopting new regulation. At the federal level, in December, the US Department of Treasury released a comprehensive report on the “uses, opportunities, and risks of artificial intelligence in the financial services sector.” The report concludes with a lengthy discussion on policy considerations for regulatory frameworks and legislative efforts, noting that there are concerns surrounding conflicting state laws and uneven requirements on AI developers, users, and financial services firms of varying sizes.
- Organizations may be subject to existing data privacy and cybersecurity laws applied to AI in new and potentially unexpected ways. For instance, organizations may not be aware of how their new AI models are exposing them to potential data privacy liabilities. Similarly, an organization may run afoul of long-standing and well-established anti-discrimination employment laws, if the company relies on biased or discriminatory AI-generated results, such as a heavy reliance on English-language Large Language Models, age, race, and other demographic features for its employment decisions.
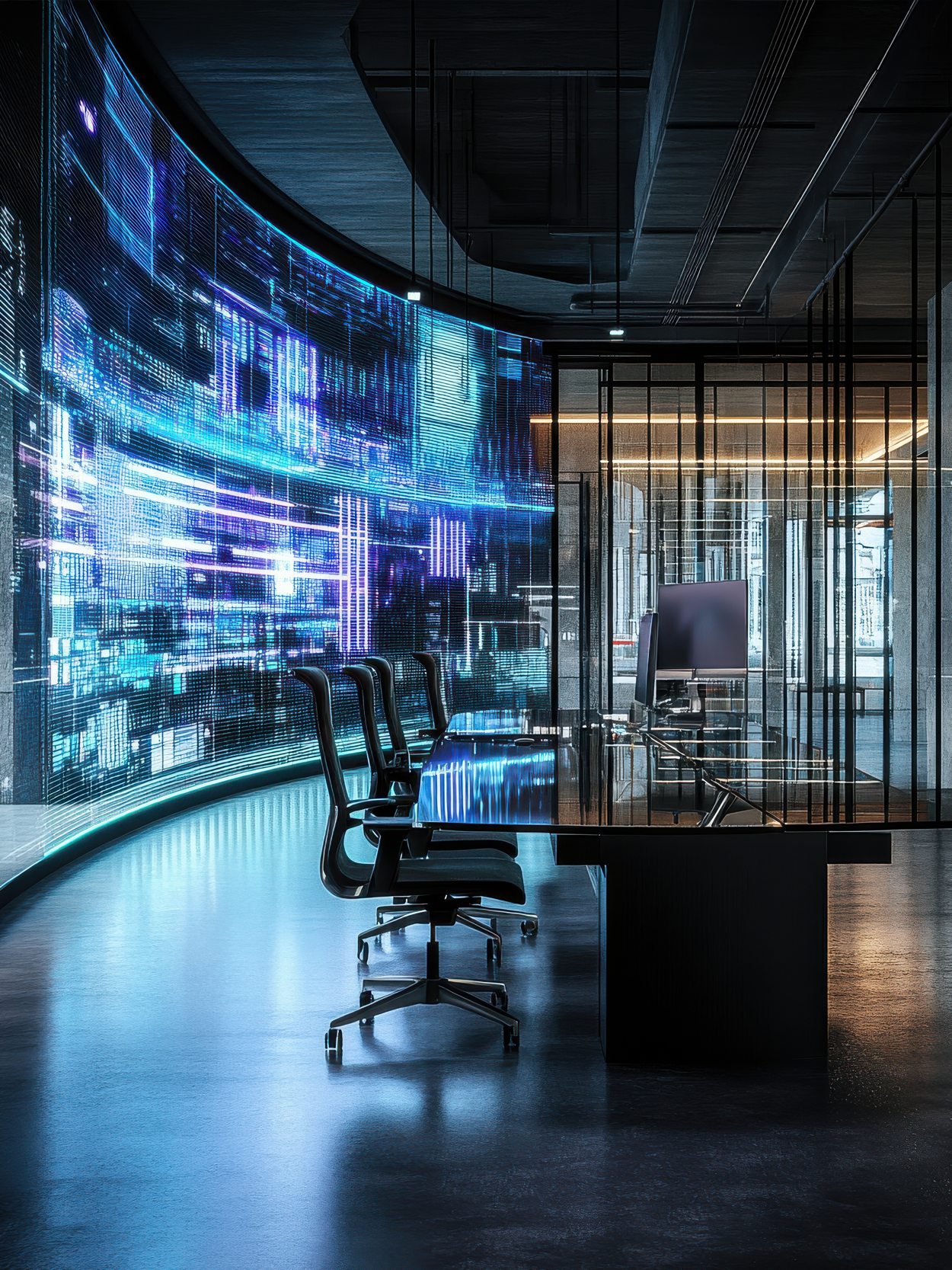
Regulatory agencies and experts around the world have raised concerns
Gen AI can produce inaccurate results that users may not be able to identify as inaccurate
AI is developing with lightning speed, and these applications rely on probabilities. When AI models yield false results, inaccuracies, or hallucinations that are not easily identified as such, the risks of liability and reputational damage increase. The Swiss Financial Market Supervisory Authority (FINMA) identified these concerns in its 2023 Risk Monitor: “Decisions can increasingly be based on the results of AI applications or even be carried out autonomously by these applications. Combined with the reduced transparency of the results of AI applications, this makes control and attribution of responsibility for the actions of AI applications more complex. As a result, there is a growing risk that errors go unnoticed and responsibilities become blurred, particularly for complex, company-wide processes where there is a lack of in-house expertise.”
Poor quality of Gen AI data can present risks of bias and discrimination
When AI relies on incomplete data sets, it can yield biased or discriminatory results, which may be a cause for concern when AI is used to make consumer-facing decisions. Even with complete data sets, AI used in consumer finance has the potential to exacerbate biases, steer consumers toward predatory products, or “digitally redline” communities, as highlighted in the December 2024 report from the US Department of the Treasury. Financial services firms that use chatbots to interface with customers should be mindful about potential liability and reputation risks as a result of inaccurate, inconsistent, or incomplete answers to questions or concerns.
Modern AI is often probabilistic, lacking explainability and resulting in opaque decision-making
AI can be either deterministic or probabilistic. Deterministic AI functions follow strict rules to render an explainable outcome. However, modern AI is probabilistic, meaning that—even for the same input—the AI may generate different outputs based on probabilities and its weights. This makes the output of probabilistic AI difficult to predict or explain. Because some laws and guidelines require organizations to explain why an adverse decision was made—such as credit decisions or insurance outcomes—if organizations can’t explain the outcomes of their AI models, they may be exposing themselves to significant liability.
Regulatory agencies, including ESMA, have identified concerns about the potential impact on transparency and the quality of consumer interactions, especially when GenAl is deployed in client-facing tools, such as virtual assistants and robo-advisors. Because service providers remain the owner of the algorithm and models, users quite often lack access to the source of the data used to train AI. When errors in data yield inaccurate results which are then used to train the AI system, the output can be inaccurate as well.
Organizations in the financial industry face concentration risks
Depending on the AI system and how it is used by the financial institution, AI tools could be considered Information and Communication Technology (ICT) assets. This could bring them into the scope of new EU cybersecurity rules applying in the finance sector, the EU’s Digital Operational Resilience Act (DORA), which starts applying on January 17, 2025. To mitigate the potential for industry-wide risks, DORA establishes certain new cybersecurity management, reporting, testing, and information-sharing requirements for organizations, which will likely have an impact on AI tools used in the financial industry. DORA requires financial institutions to assess concentration risks. Because AI models are concentrated among relatively few suppliers, the rise of third-party AI could have implications for the concentration risk of financial institutions.
AI and emerging technology inherently involve data privacy and cybersecurity risks
Because AI systems rely in some cases on processing personal information, these tools may already be subject to existing data privacy laws. For instance, some US privacy laws require organizations that use automated technology to make important automated decisions (e.g., financial and lending, insurance, housing, education, employment, criminal justice, or access to basic necessities) to allow individuals to opt out of the automated decision-making tool. US privacy laws also require organizations to (a) provide a transparency notice to individuals before using personal information in connection with the development or deployment of AI, and (b) give individuals the right to access, delete, correct, and opt-out of certain processing if their personal information is used in AI.
The EU/UK General Data Protection Regulation (GDPR) also creates strict requirements when individuals are subject to decisions based solely on automated processing, including profiling, which produce legal or similarly significant effects, along with similar transparency and privacy rights like US privacy laws. The GDPR further requires companies to document a “lawful” basis for using an individual’s personal data in connection with AI. Complying with these requirements may be overly challenging with certain AI systems, such as those that use probabilistic decision-making tools.
Additionally, widespread use of AI may lead to broadened cybersecurity risk. GenAI can be used to enable convincing and sophisticated phishing attempts that lack the usual markers of an unsophisticated attempt, including grammatical, translation, and related language errors. Specifically, password-reset requests and other spoofing and social engineering techniques used to acquire access to systems will likely become more difficult to detect, regardless of the level of sophistication. The benefits of AI-enhanced software development and other cyber operations are also likely to accrue to the most sophisticated of threat actors, including nation state actors, who have the financial wherewithal to leverage the quickly changing technological environment, increasing the risk to the financial services sector—already an attractive target.
How an Enterprise Risk Mindset Approach Can Mitigate AI-Related Risks
An enterprise risk mindset approach to AI and other emerging technology requires certain best practices.
Increase awareness within the organization
Although AI is a complex technology, organizations should ensure that their employees have a basic understanding of where and how AI is used in the organization, potential shortcomings, and risks of AI systems, how to spot inaccuracies, and prohibited uses of AI. Organizations should also identify those individuals who can answer AI-related questions and to whom employees can bring concerns.
Create a diverse, interdisciplinary team dedicated to addressing AI risks
Managing the risks and opportunities associated with AI is far too monumental for one person or department in the organization. Instead, organizations should assemble a dedicated AI team that includes stakeholders and employees with skillsets such as law, data privacy, intellectual property, information technology and security, human resources, marketing and communications, and procurement. Relying on internal and external experts and resources, this AI team should create, implement, and maintain a reliable AI governance program. The AI team should review AI-related tools (including those developed by third parties), processes, and decisions by considering risk factors associated with opaqueness or a lack of clarity, bias or discrimination, inaccurate information, privacy, cybersecurity, and intellectual property—among others.
Incorporate governance guardrails
Organizations should take steps to implement and communicate policies regarding the development or use of AI to all employees within the organization. These guardrails should reflect the key risks identified relating to the development and use of AI. Additionally, specialized or focused training guardrails may be required for specific departments or functions within the organization. For instance, organizations can instruct employees not to enter personal data or sensitive business information into AI tools and/or to only use company-approved AI systems, which have appropriate contractual protections for the company’s data.
Regulations set different obligations depending on the role of the organization and the level of risk of the AI system (risk-based approach). Organizations should determine the level of risk posed by the AI system and the organization’s role in connection with AI (e.g., developer vs. deployer), and then assess each AI system to ensure they comply with the organization’s role-specific legal obligations, and that risks are adequately mitigated. Organizations should document an AI impact assessment reflecting that the development or deployment of AI is justified, based on the risk-mitigation measures in place.
Apply a robust review process
Organizations remain responsible for the actions taken by AI systems and AI-generated results. Ignorance may not be an excuse for liability, nor is the fact that a third party created the AI system. AI systems should be viewed as a supportive tool for the organization and its professionals; AI is not an actual decision-maker. Therefore, the organization’s AI team should develop decision-making processes, oversight responsibilities, and implementation criteria for AI systems that consider components such as anti-money laundering, business continuity, communications, personal data protection, cybersecurity, risk management, regulatory requirements, and vendor management.
Establish and maintain open lines of communication with regulators and stakeholders
Numerous financial regulatory agencies—including the United Kingdom’s Financial Conduct Authority, European Securities and Markets Authority, Swiss Financial Market Supervisory Authority, Germany’s BaFin, and the US Securities and Exchange Commission and FINRA—have released guidance to help financial organizations navigate and mitigate the risks of AI. Organizations should stay abreast of the regulators’ guidance and consider engaging with them to better understand the changing AI landscape.
According to a primary research by FTI Consulting, AI practice disclosure in industry-standardized financial reporting (e.g. Proxy Statements, Corporate Sustainability Reports, or 10-Ks) should be another key consideration. For publicly traded financial organizations with reporting obligations, proactively disclosing AI practices within the organization not only shows good governance, transparent and robust AI disclosures are also a great strategic communications tool to engage with investors and other stakeholders on overall AI strategy, AI risk mitigation and highlight organization competitiveness in a rapidly evolving AI landscape.
AI Risk Management Requires a Vigilant and Holistic Approach
AI and other emerging technologies are rapidly evolving, and organizations must continually balance AI’s risks with its benefits. This is not a one-time decision, but an ongoing practice. Similarly, staying informed of the technology, its functionality, its risks, and its benefits is far too expansive for one person or department to handle alone; it requires input across functions and departments within the organization, as well as consultation with a team of trusted experts in IT, legal and regulatory compliance, communications, and governance. A holistic and ongoing approach to AI risk management will enable organizations to harness AI’s benefits while minimizing the risks of liability, reputational damage, and regulatory scrutiny.
Additional Authors from FTI Consulting
Meghan Milloy, Managing Director
Matt Saidel, Managing Director
The views expressed herein are those of the author(s) and not necessarily the views of FTI Consulting, Inc., its management, its subsidiaries, its affiliates, or its other professionals.
FTI Consulting, Inc., including its subsidiaries and affiliates, is a consulting firm and is not a certified public accounting firm or a law firm.
FTI Consulting is an independent global business advisory firm dedicated to helping organizations manage change, mitigate risk and resolve disputes: financial, legal, operational, political & regulatory, reputational and transactional. FTI Consulting professionals, located in all major business centers throughout the world, work closely with clients to anticipate, illuminate and overcome complex business challenges and opportunities. www.fticonsulting.com